In industry and culture, artificial intelligence (AI) and associated technologies are being increasingly widespread in health care. These innovations are capable of changing multiple areas of treatment for patients as well as logistical procedures of providers, payers, and pharmaceutical firms.
A variety of scientific trials have also been carried out to show that AI can do well and better than humans at core healthcare activities such as disease detection. Algorithms now outweigh radiologists to diagnose malignantly and lead researchers in constructing populations for expensive clinical trials.
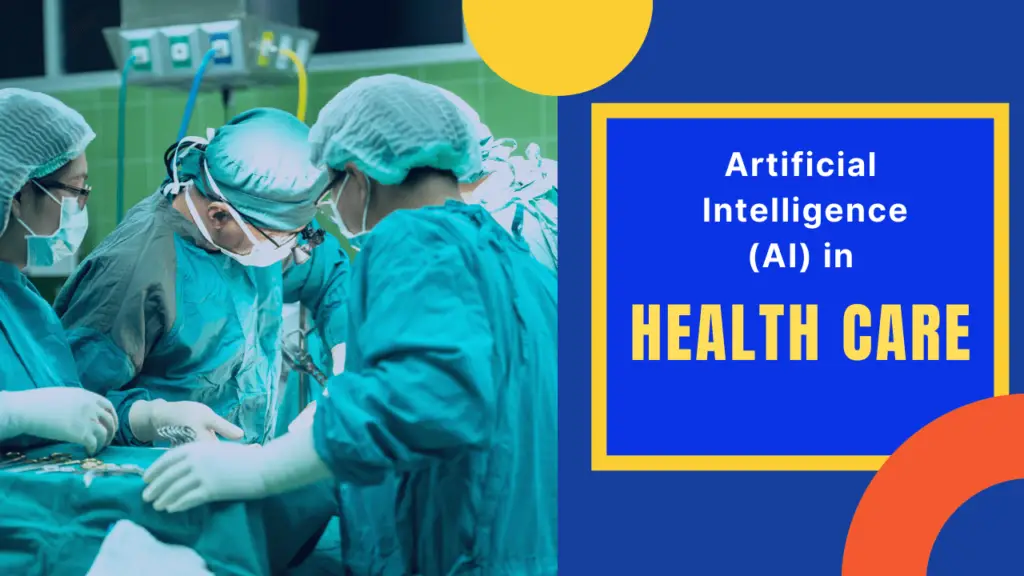
AI Types Related To Health Care:
Not a technology, but a set of artificial intelligence. Any of these innovations are of urgent significance in the area of health care, but they differ greatly in their special processes and activities. The following are described and explaining those AI technologies which are of high significance to healthcare.
Machine Learning — Deep Learning and Neural Networks:
Machine learning is a computational methodology for data adaptation and ‘learning,’ by data training models. One of the most popular types of AI is machine learning, where 63% of the companies studied used machine learning in their industries in the 2018 Deloitte Survey of 1,100 US administrators, whose organizations have already been conducting AI.
Precision prescription – the treatment procedures a patient can use based on various patient characteristics and the treatment background is the most common use of conventional machine learning in healthcare. In the vast majority of machine learning and accuracy medicine systems, training knowledge about which variable outcome (eg initiation of the condition) was defined is needed.
Deep learning is also increasingly used in the recognition of language and as such, is defined below in a type of natural language processing (NLP). Unlike previous modes of statistical research, a human analyst usually has no relevance in any element of a deep learning model. This can lead to a very complicated or unlikely understanding to describe the results of the model.
Natural Language Processing:
The aim of AI researchers since the 1950s is to establish a sense of human language. The area of language processing, text interpretation, translation, and other language-related objectives are included in this field, NLP has two foundational approaches: mathematical and semantics NLP. The NLP statistics are focused on machine learning (particularly on neural deep learning) and have helped recently to improve recognition accuracy. It takes a broad ‘corpus’ or body to acquire a language.
In the field of healthcare, NLP is primarily used to develop, understand, and identify clinical papers and published studies. The NLP systems can search unstructured patient notes, write documentation, transcribe patient-specific experiences, and perform conversational AI. NLP systems can scan patient interactions.
Rule-Based Expert Systems:
The prevailing technology for AI in the eighties was expert systems based on sets of ‘if then’ rules, and they were used extensively commercially in the later century. In the last couple of decades in healthcare, they have been extensively used for clinical decision support’ purposes and are still commonly used. Today, many EHR providers provide their devices with a set of rules.
Expert programs involve a set of rules in a specific information area from human experts and expert technical engineers. They work very well and are easy to comprehend. However, the rules tend to break down when there is a huge number (usually over several thousand) and when rules clash. Also, modifying the rules can be challenging and time-consuming if the information domain varies. More methods focused on data and machine learning models are replacing steadily in healthcare.
Physical Robots:
At this point, physical robots are recognized as more than 200,000 manufacturing robots are deployed around the world every year. They conduct preset duties such as raising, repositioning, welding, packaging, and distribution of materials in hospitals. In modern years, robots are more co-operating with humans and easier to practice in the desired mission. They are even smarter since other AI features are embedded in their ‘brains’ (really their operating systems).
Surgical robots, first approved in the USA in 2000, provide surgeons with ‘Superpower’ to improve their capacity to see, to deliver reliable, minimally invasive incisions, stitch injuries, and so on. Gynecological surgery, prostate surgery, head, and neck surgery are traditional procedures.
Diagnosis and Treatment Applications:
Since at least during the 1970s, when MYCIN had been developed at Stanford to detect blood bacterial infection, diagnosis and treatment of the disease have been a major focus of AI. These and other early regulatory structures had been promising to reliably diagnose and treat disease but were not approved for clinical practice.
In recent times, IBM’s Watson gained substantial media coverage for its emphasis on accuracy medicine, particularly cancer diagnosis and treatment. Watson uses a mix of NLP and deep learning. But the early hope for this technology implementation vanished when clients learned that it was difficult for Watson to teach Watson how to cope with forms of cancer and to incorporate Watson in treatment procedures and systems.10 Watson is no single product but a series of ‘cognitive resources’ delivered by application programming interfaces (APIs), including voice, vision, and machine learning-based data.
Applications for Administration:
In healthcare, there are also a wide number of logistical applications. Compared to medical care, the use of AI is much less innovative in this area, but it does offer significant productivity. For example, the average US nurse spends percent of the time spent on regulatory and logistical activities. The most possible technology, for this reason, is RPA. They can be used for a range of healthcare purposes, including the filing of claims, clinical documentation, control of the sales cycle, and patient records.