R&D involves the development of new drugs that are effective against a specific target involved in the disease. This involves a lot of predictive models, experiments, and expertise, each with modifications. Artificial intelligence is transforming the pharmaceutical industry. AI is automating many parts of the process of drug discovery. Moreover, statistical models and analytics reduce trial and error in drug discovery. AI could be used in the development of drugs that could be worth millions.
To derive maximum benefit from AI, businesses are searching for how it can deliver across several parts of the discovery process. Here are some of the applications of AI in drug design.
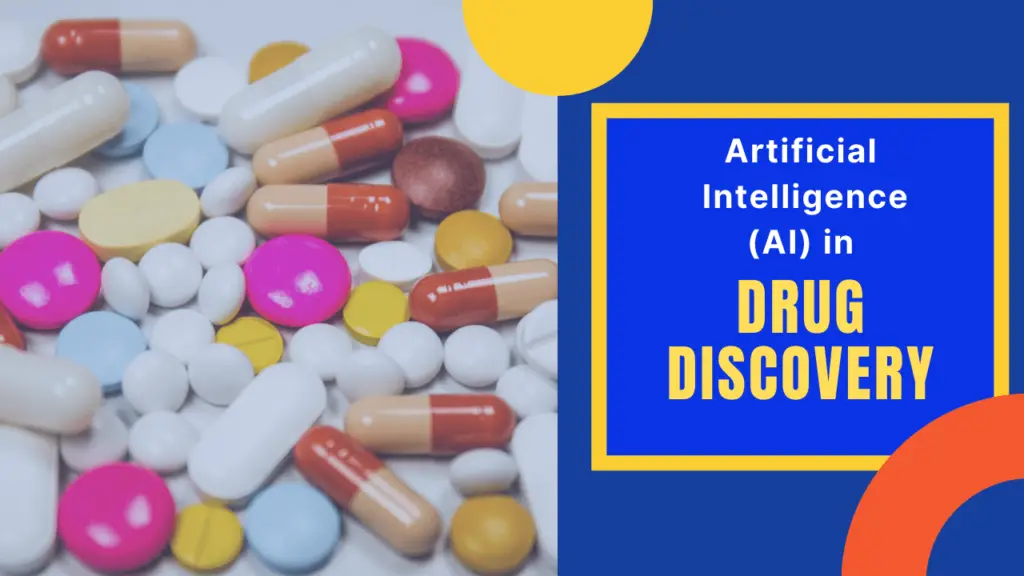
Applications of AI in Drug Design
- With AI, target identification can be achieved. AI can be employed for identifying the biological entities that need to be targeted. For instance, AI and NLP( Natural Language Processing) may be used to scan huge volumes of literature as well as genetic datasets to search for hints about gene-disease associations to identify new targets. Clues from previous literature can also tackle this. A company processing an idea should scan the past literature to check if related ideas have already been tried. This would help the company focus its direction of research.
- After the identification of the target, AI can be employed for molecular generation right from scratch. AI may be used for identifying new molecules as well as performing virtual test cycles. Although AI is a bit far from creating new drugs without any human guidance, it holds great value in bringing focus to the process of idea generation.
- AI can easily perform in silico property prediction for identified candidates. This enables the early elimination of poor candidates and also increases the throughput of high-quality leads. In-silico modeling is getting improved with greater data and improved algorithms.
- Neural networks may be used to predict retrosynthesis routes, thereby assessing a candidate’s synthesis ability. This is how it helps to comprehend how easy it is to make a drug. This greatly helps improving planning as well as eliminates avenues that would not scale viably.
- AI is used across the drug lifecycle from production process optimization to collection of clinical data to the assessment of variations in populations that can impact response rates.
- AI uses complex algorithms and machine learning for extracting useful information from a big dataset. For instance, an RNA sequencing dataset can be employed for the identification of genes whose expression correlates with a given cellular condition.
- AI can even be used for the identification of compounds that bind to ‘undruggable targets’ that are proteins whose structures are not yet identified. A predictive set of compounds can be identified in a small amount of time via iterative simulations of interactions of varying compounds with small pieces of a protein.
Artificial intelligence approaches to create new drugs
A common approach to designing drugs is to use a description of what one wants a molecule to look like. For instance, the reduced graph description which involves specifying what structure the molecule must have in chemistry terminology. This description is beneficial since it restricts the search for molecules to only those which meet the particular criteria. It is easy to create a reduced graph for a known molecule. What is challenging is to find potential molecules that match the desired reduced graph. AI greatly helps with the challenge of producing a set of candidate molecules from a reduced graph description. This problem is related to another AI challenge which is translating languages.
Language translation takes advantage of sequence-to-sequence learning and attention mechanisms which are the two main developments in neural networks. This enables translations in which the right words are selected and also captures the overall meaning. It is possible to represent a molecule as a text sequence by using a SMILES string. A similar approach applies to the reduced graph that captures the outline of what the molecule should exactly look like. We formulated an approach that applied the same principles of language translation to translate the molecule outline into a new molecule that matches the outline or in simple words to predict a molecule that meets our requirements. All that was needed was a dataset with many thousands of molecules and their equivalent reduced graph outline for training the AI system. Luckily, big datasets of molecules are available, and it is easy to generate descriptions of an entire molecule. AI may propose novel molecules for any given reduced graph that matches the specification, and which can be used by chemists to guide their quest for the next drug candidate.
Characteristics of AI-based drug discovery methods
The main characteristics of AI-based drug discovery procedures are:
- Data-driven methods.
- Complex algorithms and machine learning may extract beneficial information from a huge dataset.
- AI enables the identification of a predictive set of compounds in little time and at a quarter of the cost of traditional procedures.
Benefits of application of AI to drug discovery
The application of AI to drug discovery has several benefits which are as follows:
- AI does not depend on predetermined targets for drug discovery. Hence, subjective bias and existing knowledge aren’t a factor in the process of drug development.
- AI uses the latest advances in technology for developing state-of-the-art algorithms for drug discovery. AI can level the playing field in drug development with the rapid increase in processing power as well as the reduction in processing cost.
- AI has greater predictive power for defining useful interactions in a drug screen. Hence, by designing the parameters of the specific assay carefully, it is possible to reduce the potential for false positives.
- AI has the remarkable potential to move drug screening from the bench to a virtual lab, where results of a screen can be achieved with improved speed and promising targets can be shortlisted without any requirement for massive experimental input and manpower hours.
Conclusion
AI holds great opportunities for the pharmaceutical industry. A future AI will hold within its databases comprehensive knowledge about biology, genes, and chemical interactions. It will be capable of specifying the synthesis of candidate molecules, gathering test data, and refining further.