A decision tree is a supervised machine-learning algorithm. A decision tree can be used for classification and regression. It is mostly used for classification tasks. It looks like a tree structure. We can quickly implement a Decision Tree in Python using the Sklearn library. You have to follow the given steps to implement the decision tree classifier.
Step 1: Import the libraries
import seaborn as sns
Step 2: Import the iris dataset
iris_data = sns.load_dataset("iris") print(iris_data.head())
sepal_length sepal_width petal_length petal_width species 0 5.1 3.5 1.4 0.2 setosa 1 4.9 3.0 1.4 0.2 setosa 2 4.7 3.2 1.3 0.2 setosa 3 4.6 3.1 1.5 0.2 setosa 4 5.0 3.6 1.4 0.2 setosa
Step 3: Split the dataset into the Training set and Test set
from sklearn.model_selection import train_test_split X = iris_data.iloc[:, :-1].values y = iris_data.iloc[:, 4].values X_train, X_test, y_train, y_test = train_test_split(X, y, test_size = 0.25, random_state = 0)
Step 4: Feature Scaling
from sklearn.preprocessing import StandardScaler sc = StandardScaler() X_train = sc.fit_transform(X_train) X_test = sc.transform(X_test)
Step 5: Fitting Decision Tree to the Training set using Sklearn
from sklearn.tree import DecisionTreeClassifier model = DecisionTreeClassifier(criterion = 'entropy', random_state = 0) model.fit(X_train, y_train)
Step 6: Prediction on the Test set
y_pred = model.predict(X_test)
Step 7: Accuracy on the training set and test set
from sklearn.metrics import accuracy_score print("Accuracy on training set: ", accuracy_score(y_train, model.predict(X_train))) print("Accuracy on test set", accuracy_score(y_test, y_pred))
Accuracy on training set: 1.0 Accuracy on test set 0.9736842105263158
Step 8: Confusion Matrix
from sklearn.metrics import confusion_matrix c_matric = confusion_matrix(y_test, y_pred) print(c_matric)
[[13 0 0] [ 0 15 1] [ 0 0 9]]
Step 9: Plot Decision Tree
from sklearn.tree import plot_tree import matplotlib.pyplot as plt plt.figure(figsize=(15, 10)) plt.title("Decision Tree of Iris Dataset") plot_tree(model, filled=True) plt.show()
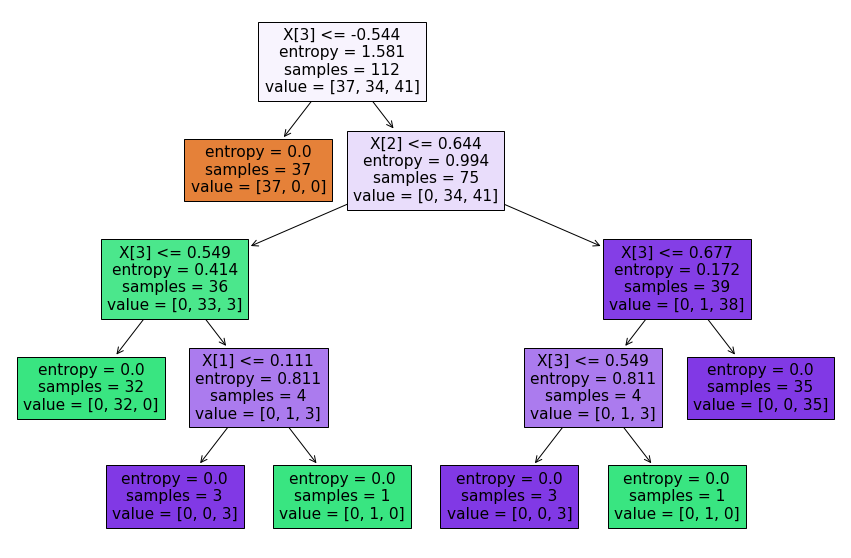
Complete Code:
# Step 1: Import the libraries import seaborn as sns # Step 2: Import the iris dataset iris_data = sns.load_dataset("iris") # Step 3: Split the dataset into the Training set and Test set from sklearn.model_selection import train_test_split X = iris_data.iloc[:, :-1].values y = iris_data.iloc[:, 4].values X_train, X_test, y_train, y_test = train_test_split(X, y, test_size = 0.25, random_state = 0) # Step 4: Feature Scaling from sklearn.preprocessing import StandardScaler sc = StandardScaler() X_train = sc.fit_transform(X_train) X_test = sc.transform(X_test) # Step 5: Fitting Decision Tree to the Training set from sklearn.tree import DecisionTreeClassifier model = DecisionTreeClassifier(criterion = 'entropy', random_state = 0) model.fit(X_train, y_train) # Step 6: Prediction on Test set y_pred = model.predict(X_test) # Step 7: Accuracy on training set and test set from sklearn.metrics import accuracy_score print("Accuracy on training set: ", accuracy_score(y_train, model.predict(X_train))) print("Accuracy on test set", accuracy_score(y_test, y_pred)) # Step 8: Confusion Matrix from sklearn.metrics import confusion_matrix c_matric = confusion_matrix(y_test, y_pred) print(c_matric)
Output:
Accuracy on training set: 1.0 Accuracy on test set 0.9736842105263158 [[13 0 0] [ 0 15 1] [ 0 0 9]]